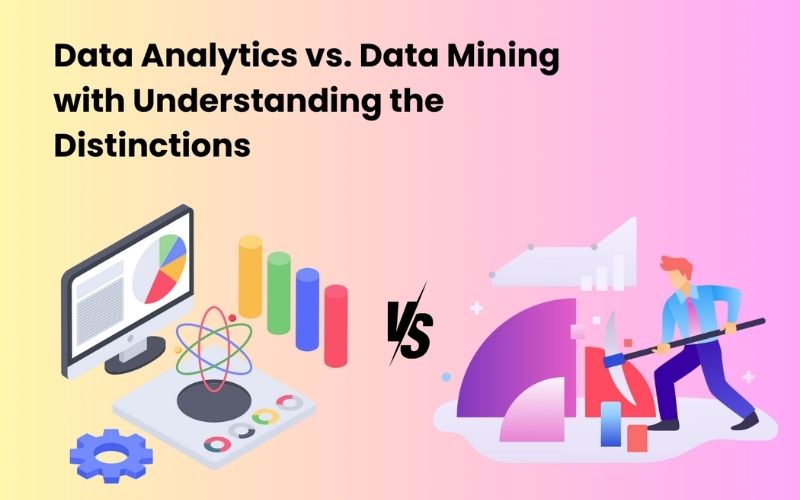
Say you have a corporation that is bursting at the seams with raw data but doesn’t know where to start. They are aware of the value buried in this sea of data, but they need to gain the knowledge to really dig in.
That’s where the importance of Data Analytics Training becomes apparent. Companies know that data may be powerful, but to fully utilise it, they must know the differences between data mining and data analytics. We will, therefore, talk about Data Mining vs Data Analytics in this blog.
Table of Contents
- What is Data Analytics?
- What is Data Mining?
- Key Differences Between Data Mining and Data Analytics
- Conclusion
What is Data Analytics?
The method of analysing, purifying, transforming, and modelling data to conclude, extract useful information, and support decision-making. It comprises analysing patterns, trends, and correlations in data by applying various statistical and mathematical techniques.
Data analytics is a type of data analytics that is commonly used in performance analysis, corporate intelligence, and market research. It works with structured data. Professionals in data analytics work with tools like Tableau and Power BI, as well as platforms like Excel, Python, and R.
What is Data Mining?
The basic purpose of data mining, a type of data science, is to find patterns, correlations, or relationships in large databases. To abstract insights, relevant information must be extracted from massive amounts of data, which are typically unstructured or semi-structured.
Data mining techniques include artificial intelligence, database systems, machine learning, and statistical analysis. In contrast to data analytics, data mining is primarily focused on identifying previously unknown patterns.
Key Differences Between Data Mining and Data Analytics
The analysts in this domain are experts in historical data, and they uncover insights through statistical analysis and visualisation techniques. Their objective is to study past trends to help firms maximise productivity, reduce expenses, and raise customer satisfaction. Conversely, data mining explores unstructured or semi-structured data from a number of sources, including text documents and social media.
Data miners use sophisticated algorithms and machine learning techniques to examine real-time data streams in order to find hidden patterns. Rather than just understanding past data, their goal is to predict future patterns, which enables firms to anticipate consumer behaviour, industry trends, and potential dangers.
At its core, data mining and data analytics offer organisations tailored approaches to effectively leverage their data, although they differ primarily in terms of data sources, objectives, and processes.
Techniques and Methods for Choosing the Right Approach
Data analytics, with its emphasis on past trend knowledge via statistical analysis and data visualisation, is the ideal tool for businesses aiming to improve client experiences and streamline present procedures. Data mining, backed by machine learning and predictive algorithms, is critical for firms looking to forecast market trends and gain a competitive advantage.
Businesses must evaluate their data sources, goals, and timeframes in order to reach an informed conclusion. While organisations working with a wide range of unstructured data sources may find that data mining’s predictive potential is quite useful, those working with structured historical data may find that data analytics is more appropriate.
Businesses can fully utilise their data by tailoring their choices to their specific needs, facilitating strategic decision-making and long-term development.
Read Also: Etiquette and boundaries in pussycat massage Prague
Future Trends in Data Analysis
Significant technical advancements will present interesting options for data analysis in the future. AI and machine learning are evolving to unprecedented levels, enabling businesses to anticipate trends and enhance customer experiences. By extracting insights from unstructured textual data, natural language processing facilitates a deeper understanding of customer opinions.
Prescriptive analytics goes beyond forecasts to suggest the optimal courses of action for desired outcomes, while deep learning is becoming more and more well-known for its ability to find intricate patterns in data.
Furthermore, maintaining user privacy and building trust depend more and more on ethical and responsible data mining practices. In the upcoming years, companies that adopt these trends will be more competitive as they will use data analysis not only as a tool but also as a strategic catalyst for innovation and growth.
Conclusion
It’s essential to recognize that data mining and data analytics are complementary in the realm of business intelligence.
These two disciplines, while distinct, work in harmony to empower organizations. Data Analytics and AI Courses can further enhance your ability to harness the power of data mining and data analytics, equipping you with the skills and knowledge needed to propel businesses into the future while gaining historical insights through data analytics.